Tech trends for 2023 and beyond
Futurice is doing regular evaluation of technologies and tech trends which might have an effect on our people and our Clients business. After some intensive rounds of interviews and workshops, the results of are in to kickstart 2023 with the latest shiny things in tech!
Global Tech Trends
Gartner has listed their Top 10 Strategic Tech Trends, which include many things that are already common practices in many organizations, but now they have new fancy names!
Besides Gartner provided tech trends and their several Hype Cycle graphs being updated continuously, there are at least two dozen Global Tech Trends going on at any given time. But we have picked few key things we consider to be relevant discussion pieces for our own and our Clients daily work in the near term.
Our top pick for a global tech trend in 2023 is Generative AI
Introduction of Open AI’s GPT-3.5 based ChatGPT in November 2022 seems to really set the expectations high for the widespread use of AI assisted software development as well as disrupting several other domains. This is the first hyped tech in a while which has sparked actual enthusiasm and application opportunities in our developer community and some people online are already labeling the technology as a Google killer.
At the same time, Dall-E 2 and similar image generation models like Stable Diffusion are proving to be worthy to produce creations that are already winning prizes in art competitions (which may or may not be deserved).
Better start learning to write good prompts! There are already open positions in LinkedIn for "Prompt Engineers".
Ongoing discussions focus on copyright, OSS licencing and overall quality issues with the generated images and code, which should be kept in mind when thinking of using these tools in client projects. At the time of writing this post, Stack Overflow has already temporarily banned the use of ChatGPT generated text. But we see these temporary bans as things that will be solved in the future as the commercial potential is huge and somebody will find a way to clarify all this legal side sooner or later.
Other global technology trends 2023
While we consider these following trends as "things featured everywhere", we do have slightly mixed feelings on how these will actually realize their hype value in the near term. WIll they actually ease the lives of the end users or bring in any of the benefits they advertise to IT departments and C-level decision makers around the globe?
Metaverse
With no clear one-size-fits-all explanation of what the Metaverse is, it has become a synonym to "We use a digital thing to boost our physical thing". A vague umbrella term to describe anything where physical meets digital. There's also the term phygital being thrown around which doesn't sound much better.
Most vocal supporter of the Metaverse concept is of course Meta, but their approach is being questioned around the world and it is not currently looking like any major new breakthrough is going to happen in the near term. Some specific areas in VR/AR domain like training opportunities for specific tasks or providing remote support have been around for a good while now and are getting more mature.
If we consider the more granular application areas like the "Industrial Metaverse", concepts like Digital Twins and Suppy Chain Control Towers are indeed important topics with great potential to boost the business of several industries, but rather than keeping the discussion on the Metaverse level, we would discuss about the actual problems worth solving and finding the best technologies to solve them with.
A "Metaverse" as-is can't magically solve any of them.
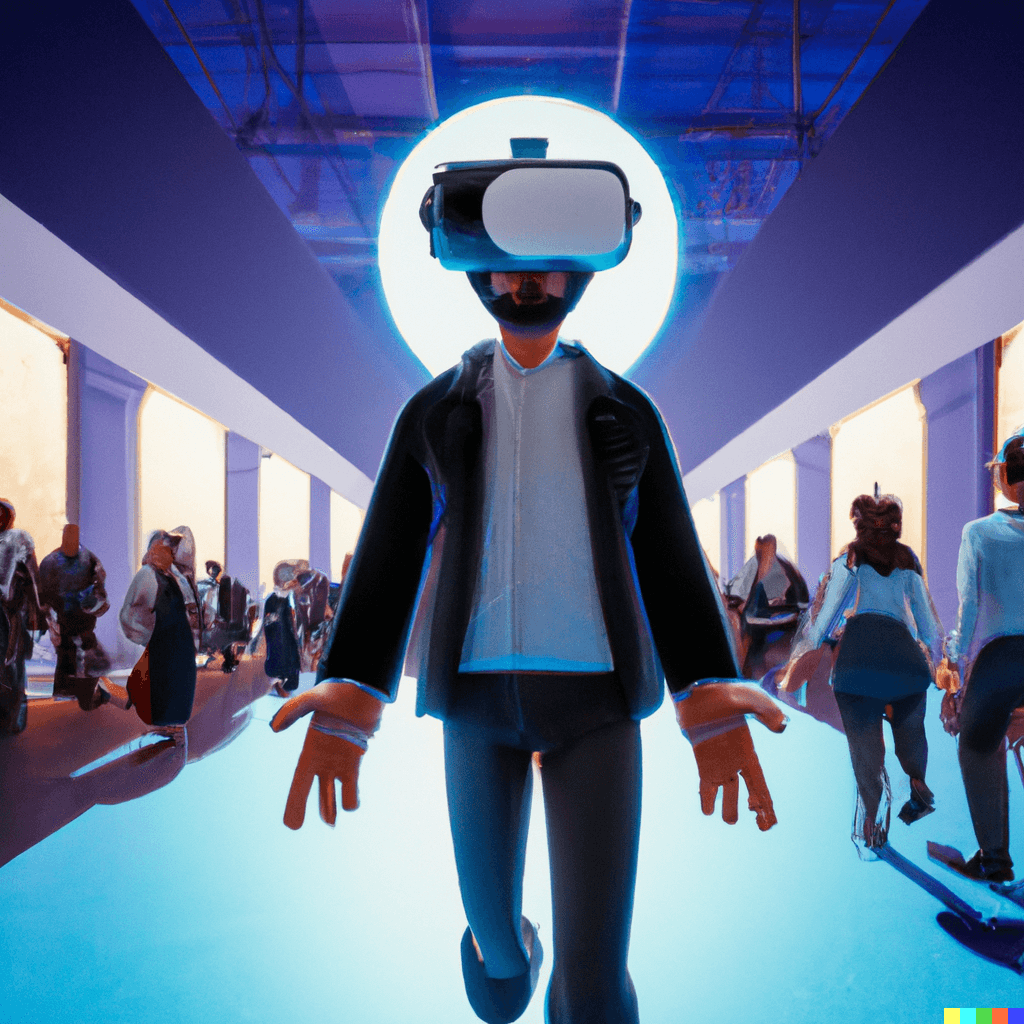
Low Code / No Code
For this trend, we focus on the "End-to-End Platforms" which offer everything from infrastructure and user management to integrated IDEs in order to build web & mobile applications using a visual editor. However utilizing a subset of the features these platforms offer is more common as several cloud platform native tools utilize the similar principles. Like you'll learn a bit later in this post.
Low code and no code platforms are being advertized and are seen in the general media coverage as a way to help in the global Tech Skills Shortage by giving non tech people ways to build things.
Altough the “citizen developer” concept is being part of low/no code marketing message, the platform vendors actually say you will need dedicated developers to build anything beyond very simple tools, closing the gap between “traditional” development and low/no code. But they are not taking away developer jobs just yet.
Platforms might cover around 80% of the requirements out-of-the-box, with 10% customization potential, but the last 10% is the tricky one (“The Last 10% Trap”).
Also these platforms usually work in quite narrow use cases and possess new challenges related to security and general governance, which we believe may result in big problems in near future for IT departments wrestling with apps built with too little oversight.
Web 3
Most of the Web3 hype is focusing on the potential of decentralization of everything, but the actual development is focusing on crypto markets and trying to make everything a sellable asset.
There are big problems with platform bugs being exploited and crypto exchanges doing so called rugpulls to defraud their customers, making any potential use cases for this technology look very unappealing or risky.
Also, in reality, there’s no “Web3” or “Web2” to choose one or the other from.
There's a good opinion piece written about Web3 (by Kailash Nadh) which describes well how the global hype where "web3" will replace something called "web2" is quite far from the truth.
Decentralization beyond “Web3” blockchain and crypto context is another topic, with platforms like Mastodon gaining some interest on the wake of Twitter mayhem. For example, there may be interesting developments around the ActivityPub protocol beyond Mastodon in the future.
In general, we see decentralization and blockchain as just another tools in the digital toolbox which can be utilized when deemed feasible. It's not an all-in type of situation.
Technologies of the Future - In our bubble
We asked our developers, data engineers, and data scientists their opinion on which programming languages and tools they think will be the winning choices for companies trying to build long-lasting digital products (which should also have skilled people available to develop them further in the future as well).
Our list is of course quite limited in scope, as we are focusing mainly on custom web applications, cloud & data platforms, and mobile development, so not all the greatest technology trends in the world have been listed here.
Also, predicting the future is hard. While doing a bit of research into our old similar exercises dating back to at least 2014, we spotted e.g. several Windows Phone related technologies listed as something to "Trial" or "Adopt", but that didn't turn out so well in the long run. Things move fast in tech and something that is popular now might disappear from the radar in a blink of an eye.
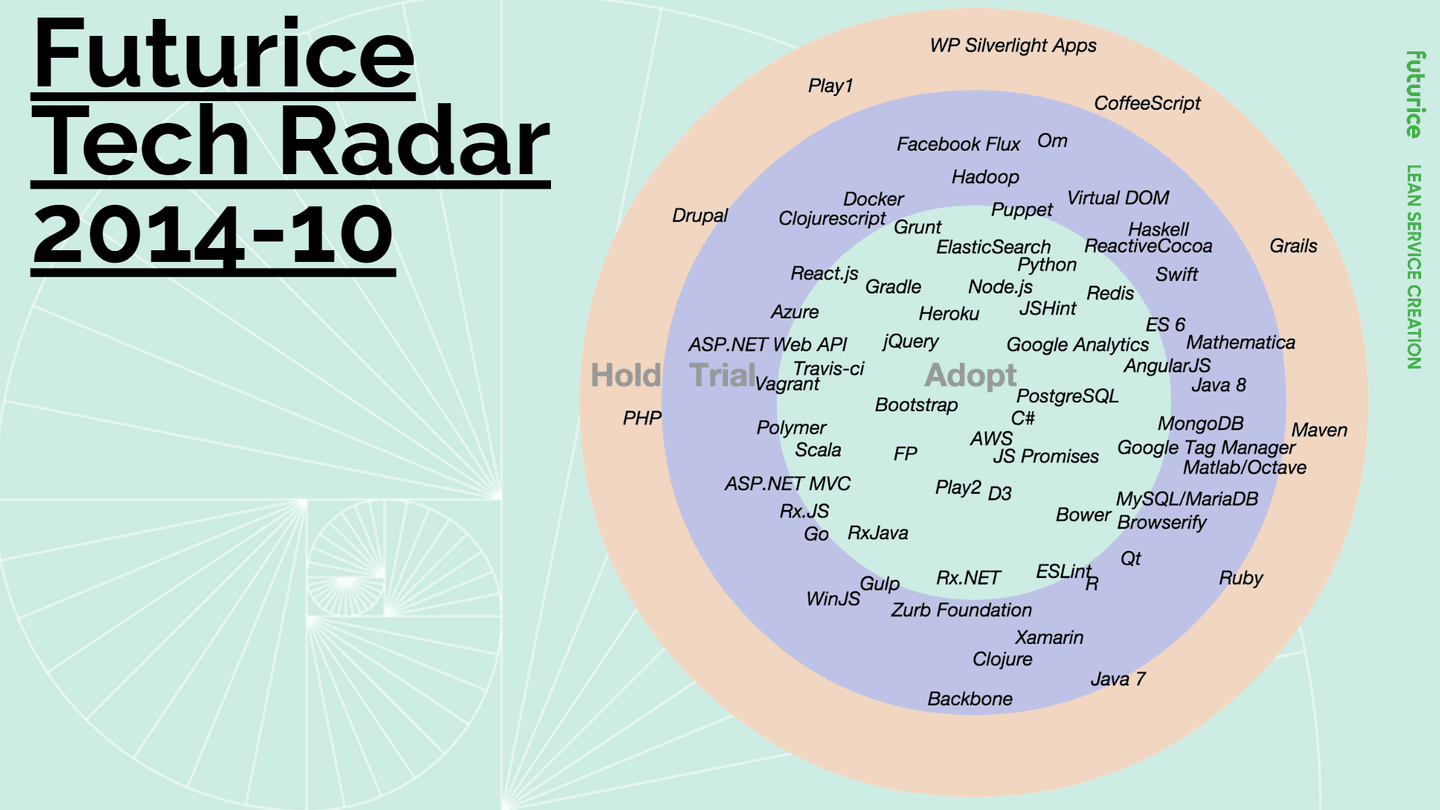
Yet, many of the predictions done back then are topping our list also this year - they have prevailed and there's a shared view they will be there for years to come.
General skills
Besides knowing the tricks of bending specific programming language to do magical things, our people highlighted several topics they feel are required now (and especially in the future) from them as members of cross-competence product teams. Paying attention to these topics when crafting our career paths and learning opportunities is even more important than getting more in-depth knowledge about a certain technology.
- Security & Privacy
- Accessibility
- Communication & Presentation skills
- Generic cloud platform basics
Especially the Security and Accessibility topics are something which tend to receive too little attention in the product backlogs across industries, when there's business-driven urgency to push out new features for the end users. However, the threat landscape featuring things like personal data breaches and DDoS attacks make securing and reinforcing the digital platforms a top priority. Threats are evolving constantly and we need to stay on top of our game.
Accessibility features on the other hand is something we consider a must-have priority even for companies not that pressured to implement them through legal requirements. It's just the right thing to do to give equal opportunities to all users.
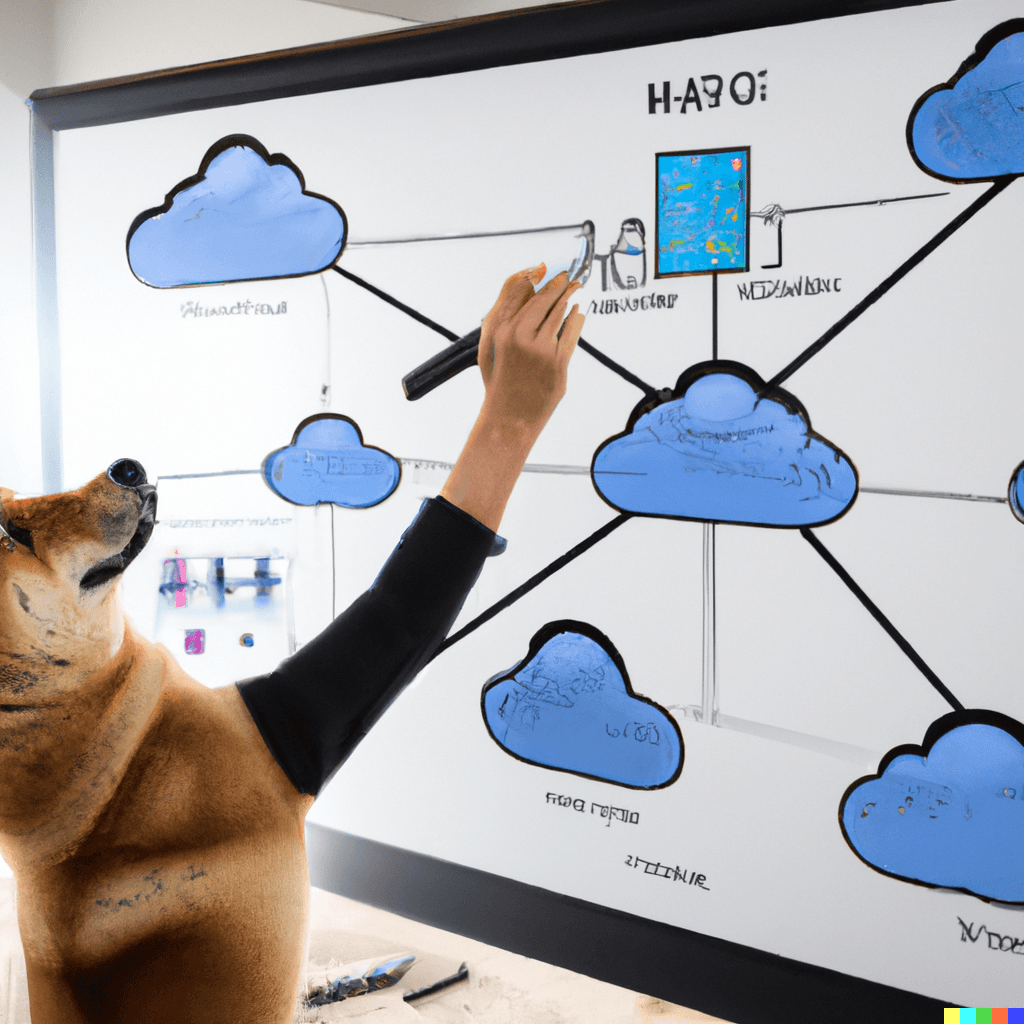
Web frontend
On the frontend tech trends side, things can get pretty boring as Typescript + React combo shares the top spot as something that has become so widely used and known by the developer community that it is seen as the key combination for the coming years. React actually appeared on our tech radar in 2014 in the "Trial" category!
This time, the following technologies were listed as the most desirable in the frontend category
- Typescript + React
- Vue
- Svelte
- NextJS
- NuxtJS
Not that many frameworks or random libraries were mentioned this time. Few random picks include Fresh and Phoenix Liveview.
Backend
On the backend, there's clearly more variety. In backend development, it is not always that critical to jump on the latest trends like flashy UI frameworks, so there are plenty of different technologies which have been around for a long time and will be in the future as well.
The current backend technologies we currently see at Futurice as future relevant are
- Typescript + NodeJS
- Golang
- Kotlin
- Python
- C#
Modern Java and Scala are not topping the lists this time, but can and will still be encountered in many places in the future. On the other hand, Elixir and Rust are seen as interesting technologies, but their current application areas are not that relevant in our work in near term.
In November 2022, we had our first Rust training organized at Futurice by our enthusiastic Rustaceans.
Mobile
Mobile development has seen a slight shift from native apps to favor cross-platform React Native, Flutter, and Progressive Web Apps (PWA). When talking about mobile apps, it's very important to always consider which type of apps are being built and select the technologies accordingly.
For simple apps, choosing a PWA might be sufficient for certain use cases, but leveraging the full range of mobile device hardware capabilities should steer towards selecting a native approach (with Swift for iOS and Kotlin for Android are the way to go). Technologies like the Ultra Wide-Band (UWB) are being introduced to mobile devices, which might boost the native apps in the future.
Currently, we are working with all of these different techonologies at Futurice and can't pick one over the other that would be the clear winner for the years to come (also our Windows Phone predictions done in 2014 didn't go that well).
Data
In data, instead of focusing on specific technologies, our data experts listed some concepts which they believe are something that can deliver business value when applied to the right contexts in the near term.
Also, in contrast to the rather pessimistic view on no code / low code platforms above, in the field of data, these are often daily tools in building data pipelines and even ML/AI solutions for faster value creation. This was something in which they believe will even grow in significance going forward.
And that's about it - this time
Wow, that was a long post! I'm glad I had the help of a generative AI with the pictures and some proofreading.
We've also shared over 50 slides of detailed information about our past, present, and future projects with our people. That's surely a light holiday novel everyone would like to get and read this year! We believe this will help guide our focus for the coming year(s) and keep us up to date with the latest trends.
I hope everyone enjoys this holiday season and looks forward to what 2023 has in store. There will be new exciting training and community events to participate in and stay current with industry trends.
Thanks for reading, and let's embrace the new year with enthusiasm!
- Lauri AnttilaAlumni