Experiences from the frontlines: How GenAI transforms customer service
In today’s fast-paced business landscape, customer service plays a pivotal role in any enterprise’s success: the ability to engage customers effectively and address their needs promptly is at the core of customer satisfaction and retention. Now with the emergence of generative AI and new capabilities with it, we are happy to share what we have learned on the frontlines and how to accelerate the customer operations transformation.
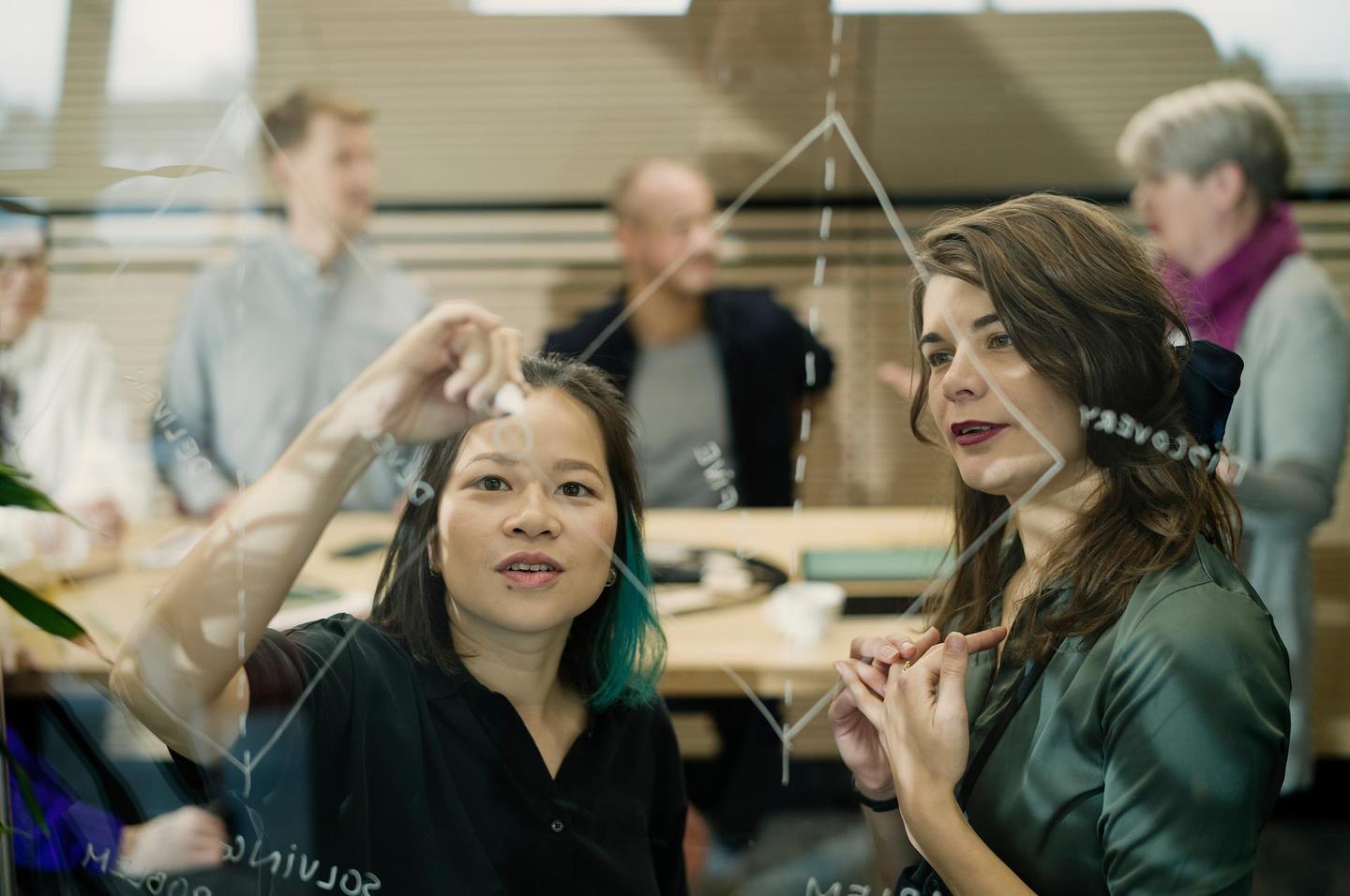
Based on a recent McKinsey study, generative AI is expected to have the highest impact on customer operations and sales processes. This is something we’ve also learned and witnessed firsthand with our clients, who we’ve worked with during 2023 in numerous Generative AI projects across different domains. Traditional customer service process phases could be simplified into 1. Customer inquiry, 2. Case categorization, 3.Handling and Resolution, and 4. Continuous analysis, on how to improve the CX and service. We’ve discovered that regardless of the industry and products or services, certain problems - high volumes, complex system landscape, manual steps, limited visibility - are generic and affect the whole value chain. Let’s dive deeper into what these opportunities mean for the (near)future of customer service.
Self-service will improve significantly and enable the solving of more complex customer inquiries
Firstly - the one that everyone talks about - the role of self-service is undoubtedly going to expand. The infuriating, clumsy experience of dealing with automated rule-based chatbots & voice agents will soon be a thing of the past. And they’ll be replaced by a new wave of both powered by Large Language Models (LLM) that are able to meet diverse user needs via text, voice, or image inputs.
The key is the LLMs’ ability to comprehend & generate language, which gives them the power to interpret & respond to the wide range of requests customers come up with - without having to predict every possible combination of question & answer up front. Of course, this doesn’t happen with the out-of-the-box solutions alone. But with the language abilities of powerful base models like GPT-4, we can then prompt & fine-tune them to specialize on particular tasks, and augment them with domain-specific knowledge via Retrieval Augmented Generation (RAG).
As a result, the end-customers get fast and effective solutions from chatbots 24/7, in the language & mode they prefer - before needing a person’s help.
24/7 chatbots that actually work: Using chatbots as first-level customer service with multi-lingual & multi-modal (working with text, voice & image) capabilities, to resolve issues and identify the right experts to deal with more complex ones.
Self-service quality level-up: Enabling customers to explore knowledge-base articles, product policies, and website content with natural language or execute online tasks & fill forms via an LLM, prompt engineered or fine-tuned to work with your systems.
Faster case resolution with live support from AI -assistants
Even though self-service will grow, it’s not going to make customer care agents obsolete. Moreover, there are still many complex issues that require human intervention or personal touch. While AI assistants can handle straightforward queries, customer agents can shift their focus to more complicated problems or situations that require a deeper level of understanding or decision-making. Additionally, some customers still prefer human interaction, especially in sensitive situations where trust and rapport are crucial.
When human expertise is needed, we believe every customer agent will have an AI assistant to support them in navigating faster the complex environment of product information, previous customer interactions, company policies or instructions, and domain-specific knowledge. In a recent client case, we saw that agents had 8 different systems to navigate to find or update information to resolve their customer issues. This meant they were often spending minutes searching while the customer got more and more frustrated - not a good experience for either the customer or the employee.
Generative AI assistants can prepare tasks for the agents to continue: classify cases and assign them to the correct workflow, handler, or escalation path and listen to or read customer inputs - and then offer answers & recommended actions in real-time for the agent. At the same time, we are also getting quality data from the touchpoints and contact reasons for further analyses and service development. With better access to the right knowledge, agents can solve customer problems faster, escalate less, and expand the scope of their roles. They’ll also onboard faster and stay longer - when they have powerful tools to help them do their jobs better.
Case categorization: Classifying and labeling cases, guiding cases to correct workflow, and identifying escalation paths based on digital footprint data and text analytics
Finding knowledge for agents and composing answers : 360 Customer view from connecting & summarizing customer information and past touchpoints across systems. Helps navigate and condense scattered company knowledge bases, policies, guidelines & product/service information. Drafting personalized replies based on identifying & summarizing similar past cases, company policies, knowledge & past customer activity.
Unlocking new value and tackling emerging problems proactively through customer input analysis
Lastly - and this is where a big impact lies - with the help of generative AI we are able to get unstructured or “silent” data for analyses and feed that to service, product or process development or other company functions like R&D. Most companies already utilize customer feedback and product reviews to guide product development and prioritization, but there’s a lot of untapped insight that is scattered in social media or between the lines in customer service chats, emails or phone calls, that can be extracted and summarized with the help of generative AI and machine learning.
Ultimately the goal is not to only fix the problems, but to find insights that enable us to stop the problems from happening to enhance customer satisfaction and retention continuously.
The other side of the coin is to spot growth opportunities to reach out with personalized solutions or new services before customers ask for help.
- New insights into emerging problems: Analysing unstructured customer feedback and complaints, to find problems worth solving early.
- Root cause and flow analysis: Analysing call transcripts, to identify patterns related to slow resolutions, escalation & low sentiment combined with flow analytics to increase visibility of service operation flow, across customer touchpoints.
- Proactive, personalised solutions: Predicting customer needs, based on past behavior. Personalized solutions, content recommendations & upselling.
Start experimenting but keep the end-to-end transformation in mind
As we learned, there are plenty of opportunities across the customer service process to enhance customer experience and efficiency but also learn how to fix problems before they become problems. Individual use cases are where the thinking starts. With each solution, we are also building the tech foundation, understanding, buy-in, and momentum for more. Generative AI holds the potential to be a disruptive force and we should keep our eye out for the end-to-end process to enable true transformation.
So if you haven’t already - start experimenting, learning, and paving the way for a bigger transformation with the help of generative AI.
- Heidi VossPrincipal Advisor