Non-technical Skills Matter - my first year as a data scientist in a consultancy
__I joined Futurice, an innovation consultancy, as a data scientist approximately one year ago. Before the job interview, I worried mostly about having sufficient technical knowledge. They were hiring me for a technical position, so the things that should matter the most are my technical skills, right? As it turns out, not exactly. __
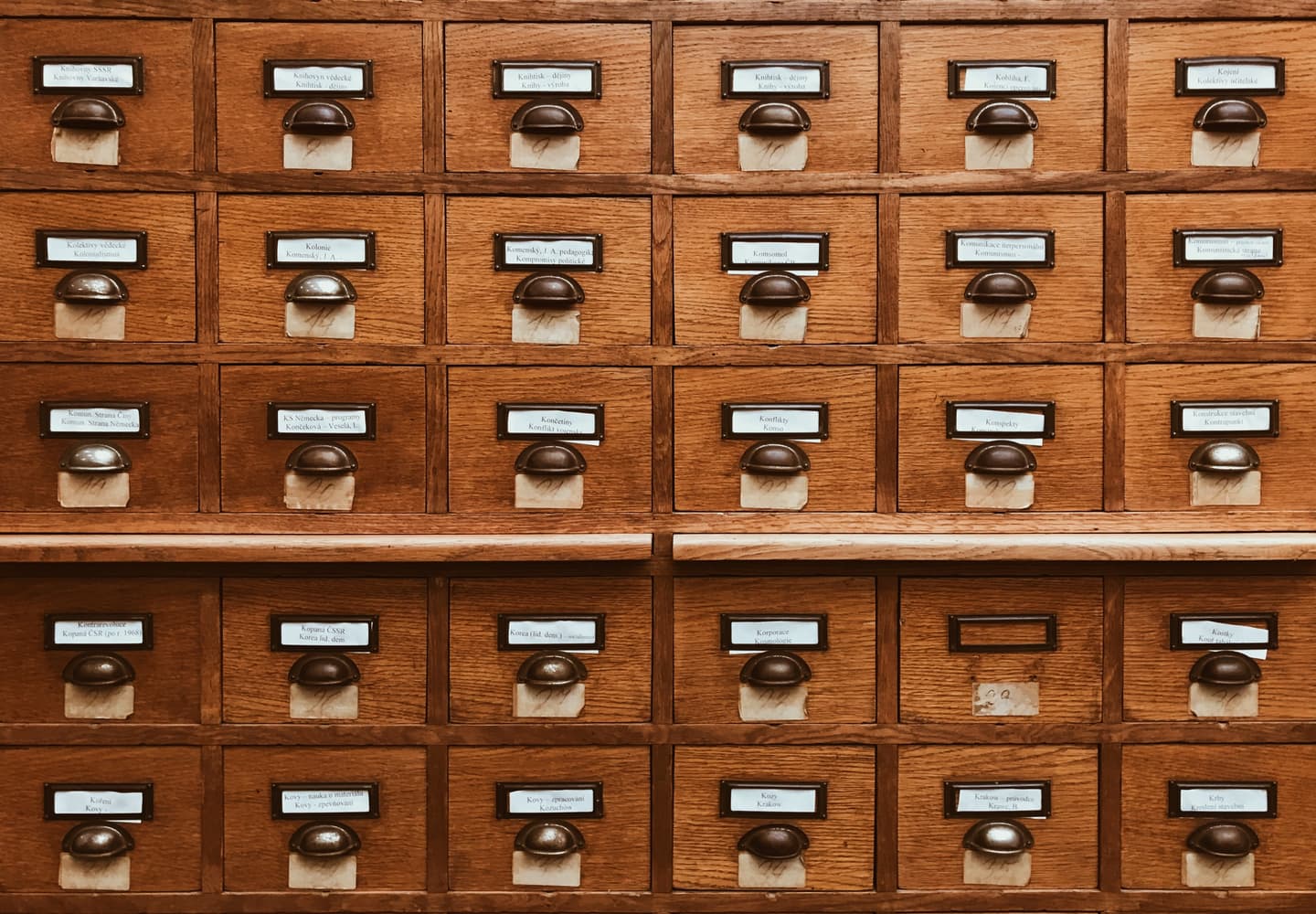
I knew that soft or non-technical skills mattered as well, but I was fairly confident I had that part covered. What I’ve found in my first year at Futurice was that besides getting to use my technical know-how in various interesting and challenging projects, I had to step up and improve a lot in non-technical areas, especially those related to understanding the sales process, consultancy business, and how to communicate with customers and colleagues alike. My perception of what are the possibilities for impact as a data scientist broadened significantly.
These lessons are from my personal experiences, both positive (e.g. I was happy to discover that I could have a positive impact in a new surprising way) and negative (e.g. I wish I had handled this issue in a different way). Your mileage may vary:
- Proactive communication is your primary risk management tool
- Aspire to be a trusted advisor to clients and colleagues alike
- Look towards solving strategic business goals of the client to increase your impact
I wanted to understand what it means to be a consultant. I wanted to understand the role of sales in our business. I read some books that are considered - at least based on Amazon reviews - essential on these topics. The books helped me clarify and distill the lessons I’ve learned so far working as a data scientist in a consultancy company. I’ve listed some of them at the end of this post. I would recommend reading those to anyone who is working in a consultancy in a technical role and aspires to work more closely with clients and business-oriented colleagues.
Proactive communication is your primary risk management tool
As cliched as it sounds, this has probably been my most valuable lesson. Usually, in a project, there is the technical and non-technical staff, with the latter being expected to take care of most non-technical issues. However, the data scientist still has the responsibility to proactively communicate about non-technical issues that might be blocking their work. Why is that? Data science projects are inherently riskier since there is more uncertainty involved in the outcome of the project. For example, you cannot know in advance whether the data that the client has will allow you to get the insights/solutions that your client expects. I strongly believe that data science projects should be handled from a risk management perspective. So it’s in the best interest of the data scientist to be vigilant about any non-technical risks that might affect their work and ultimately the success of the project. Most of those risks can be mitigated with proactive communication. Some examples:
- The client is unable to give you access to their data in a timely manner. This can happen when not all stakeholders on the client side share the same feeling of urgency for forging ahead. For example, the IT person (or whoever is your project counterpart) on the client-side who is responsible for giving you access to the data might have a lot on her table and does not prioritize this task. Explain to them what this delay means to the project schedule and the urgency of this first step. Communicate as frequently as needed with your counterpart until the issue is resolved. In the end, the data scientist/IT person from the client-side might be annoyed with you, but not as much as the client PO would be when she finds out the project has been delayed, again.
- Does the client have unrealistic expectations regarding what can be done with AI or within the available schedule of the project? Challenge them and discuss what solution fits their actual needs the best. Don’t be afraid to share your opinion (in a respectful way, of course). More often than not, you are the expert in the room and even if the client does not necessarily like it when you pop their bubble, they will appreciate your sincere effort to do the best you can to help them. If you do not manage expectations regarding the possibilities of AI or the outcome of the project, you may risk having an unhappy client even though you delivered what is in your opinion a good result.
- The client is not sure whether you are the best person to solve their problem. The best way to show your credibility is not by waving your CV and asserting that, yes you are in fact the expert, but by illustrating your knowledge. If the client already mentioned what challenges they have, then you can begin a discussion on how you would start solving them. Maybe mention your previous experience that might be relevant. In the end, you are a consultant and the best way to deliver value to the client is by getting started on solving their problem right away. The sooner you deliver value, the happier your client will be. However, if you feel that you are not the best fit for the challenge, then be honest about it and adjust the project team so that needed skills are available.
As can be seen from these examples, communication skills are not just about polite conversation and cheerful disposition, but an essential tool to proactively remove obstacles to your work and minimise non-technical risks. Data scientists are responsible for recognising and mitigating both technical and non-technical risks in data science projects.
Aspire to be a trusted advisor to clients and colleagues alike
There is a lot of hype around AI and data science, and it seems to be spreading to almost all industries. This, in turn, means more data scientists get hired and the salaries are competitive. On the other hand, an understanding of what AI actually is and what can be done with it is somewhat lacking, especially among people with a non-technical background. This leads to unrealistic expectations and missed opportunities. The latter can be especially frustrating. For example, I bet that in every company that is just starting with AI and data, there are some low-hanging fruits - problems that can be solved with relatively simple data science methods and existing data - that would provide an excellent ROI and address some important business goals. You just need people to be able to identify them.
This is where an important aspect of being a data scientist comes in - your role as an advisor and educator to your colleagues and clients alike. You can start by simply showing a welcoming attitude to your colleagues, by letting them know that you are happy to help if they have questions about AI and data. You can support salespeople when they are preparing a proposal for a client to make sure that all the bases are covered or just be generous in accepting to participate in preliminary meetings with clients to help them explore their options.
There are a lot of opportunities to help out and make a difference, and you would be surprised how your non-technical work (“emotional labour” as Godin calls it) will allow you to learn more about your business and most likely have a positive impact on the bottom line of your company. One of the most gratifying experiences I had last year was running our internal AI crash course. The goal of the course is to establish a base level of understanding about AI and data science within our company, and give people from different competences the insights that are relevant to their work. It was an interesting challenge since I wanted to make sure that everyone felt that they got something useful out of it. AI and data are complicated topics that have an impact on almost everyone’s work. As an expert on the topic, you can make a difference in people’s lives by helping them understand, which in turn will make you a valued colleague (or advisor to the client) whom they feel they can trust to give good advice.
Look towards solving strategic business goals of the client to increase your impact
There is a point-of-view that a senior data scientist is someone who not only knows the theoretical and engineering side of data science but also understands the “business” side of things. I tend to agree. The goal of a consultancy is to deliver value to clients, preferably by addressing their strategic business goals. The impact of AI and data science solutions is measured by how effectively they solve the client’s business needs. If you want to have a bigger impact as a data scientist, you need to understand these needs and figure out how to best serve them.
For example, when writing a data science project proposal, I make sure whenever possible that we are addressing a business goal with a clear business metric that tells us how much value we deliver if we succeed. Once we have a clear target on the business side, then we can translate that into data science tasks and their accompanying metrics. This ensures that our work is meaningful, serves as a great motivator to people working on the project and invites buy-in from the business stakeholders on the client-side.
On a related note, if you would like to grow as a data scientist, then besides becoming an expert on some deep technical topics, the consultancy business also provides you an excellent opportunity to do some business/strategic advisory work for the client. As one of my colleagues put it, if you have a good understanding of both the technical and strategic side of AI and data-driven organisational change, then you can consider yourself having acquired a superpower. Of course, this will take time and it also depends on your personal ambitions. For myself, I find the topics related to business strategy quite interesting and I’m looking forward to learning more.
Recommended reading
There are a lot of books written about sales and consultancy. When compiling my relatively short reading list, I tried to focus on books that were mentioned in at least a few of Top-10 lists and considered “essential” or “fundamental”. This list is by no means exhaustive but provides a good starting point for a data scientist who wants to look beyond their horizon.
The books by Miller & Heiman might be considered ancient by now, but they do provide a nice systematic (albeit quite wordy) view of the sales process and give a solid foundation for further learning. I found many great insights from the books of Lencioni and Maister on how to establish a trustful long-term relationship with a client. In the end, that is what consultancy is all about. Finally, I found Seth Godin’s book very inspiring, as it forced me to consider what is the unique perspective that I bring to my day-to-day work.
- The New Strategic Selling
- Miller & Heiman
- The New Large Account Management
- Miller & Heiman
- The Trusted Advisor
- David Maister
- Getting Naked
- Patrick Lencioni
- Linchpin
- Seth Godin
- Vilen LoogaData Scientist