Transforming B2B Sales with Generative AI - experiences & lessons learned
Since ChatGPT hit the web in 2022, generative AI has been a major focus of our client work - it’s been quite the whirlwind! B2B sales is among the processes where we’ve seen the biggest impact. The business case is often clear, when improvements in quality and speed have such a direct impact on the income statement. And there are a lot of knowledge-intensive and high-frequency tasks that suit gen AI well. So we thought we’d share some stories, on what we’ve done and what we’ve learned.
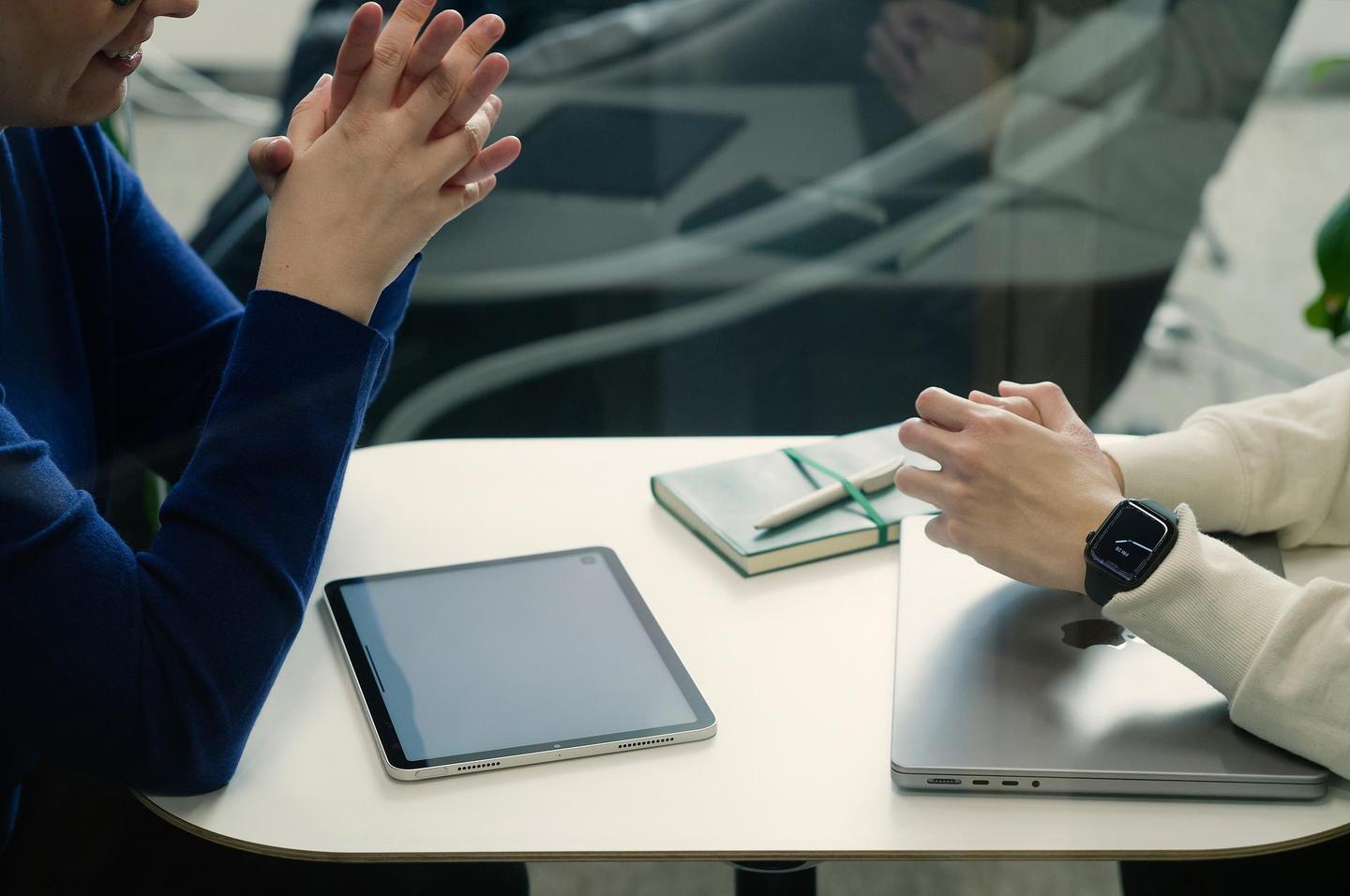
Building a knowledge-centric sales process - at Futurice!
At Futurice, we always try to walk the talk, and act as the first guinea pigs for the actions we recommend to our clients. That's been true in sales more than anywhere else.
We create around 5-10 new sales proposals & thought leadership presentations every week, which represents a whole lot of useful thinking. We also have over 1000 experts across the Futurice Family, with experience in just about every tech, business & industry domain. But most of this was grossly underutilised in our sales, because people couldn’t effectively find our best assets or experts. That meant a lot of reinventing the wheel - which increased sales time & reduced quality.
We’ve tried many initiatives to get people to manually document & tag their best sales assets in platforms like confluence (our company intranet), but it never works. When people have long lists of urgent to-dos, ain’t nobody got time for documenting knowledge that might be useful to someone at some unknown point in the future.
So we developed FutuCortex, an automated knowledge platform and expert search. It automatically identifies & extracts knowledge directly from the tools we use and the ‘digital footprint’ we create. So we have a constantly updating knowledge base, with no need for manual documentation.
This year, we’ve been experimenting with new possibilities from gen AI - and the results are rather exciting! In short, we’ve built a ‘retrieval augmented generation’ (RAG) application on Azure, which feeds GPT-4 with knowledge from a database of 10s of thousands of our past slides and docs. This gives our sales people a safe space to chat with GPT-4, and get referenced answers based on our own proprietary knowledge, rather than the black box of these models' vast training datasets.
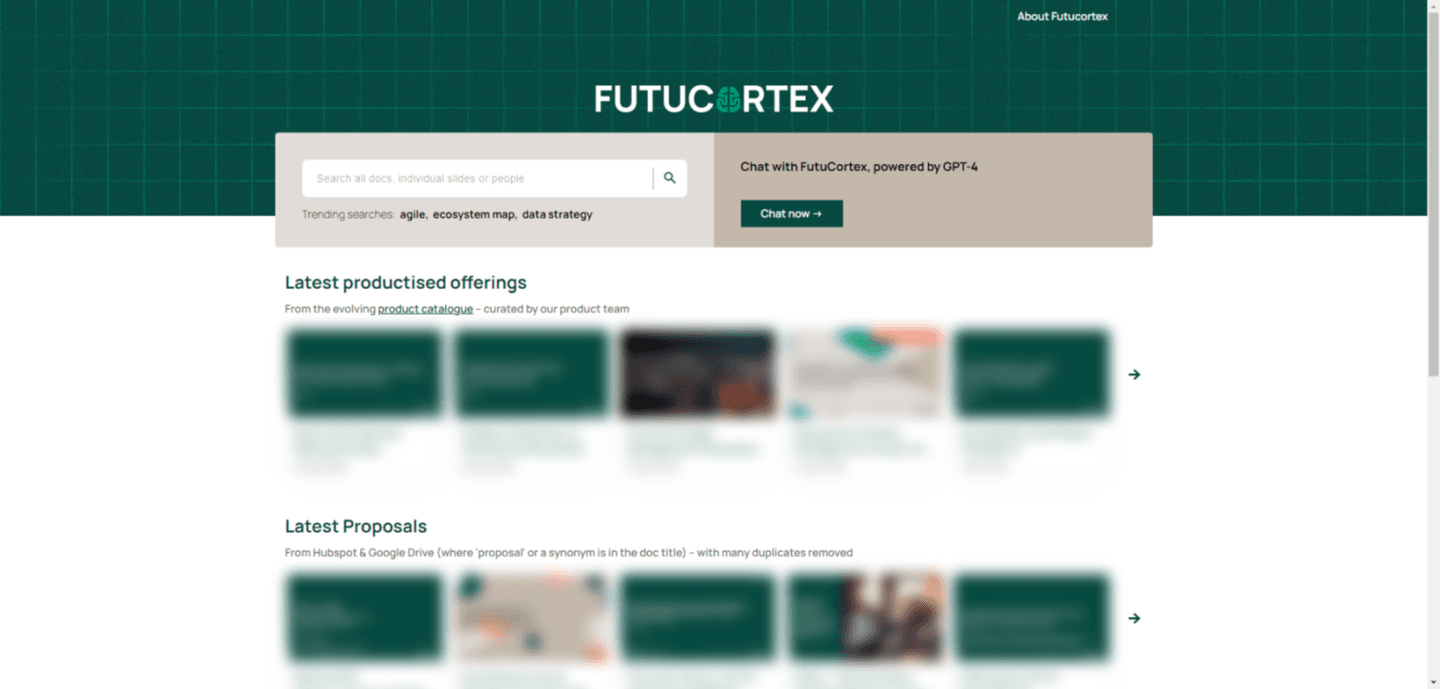
FutuCortex was already used weekly by over 50% of our salespeople - our key target users. But since releasing our gen AI features, our average unique weekly user count has more than tripled. This now includes almost all our salespeople and many others who are hacking new use cases beyond sales. Pretty nice!
To get here, we’ve learned it ain’t easy. You can make simple RAG applications really fast. But getting something that creates real value in production requires a lot more than slapping a chat interface on top of your search. The LLM answers can only ever be as good as the context you give it. So, if your SharePoint or Google Drive search results are shitty (they usually are…), adding GPT on top will only create polished-looking but still shitty answers.
So we’ve had to get familiar with the rather large toolbox of things you can do to improve the context & answer quality: document processing, metadata extraction, classification, search relevancy tuning, reranking, prompt intent recognition, query expansion, prompt engineering, and more. It’s clear there’s no one size fits-all approach. So you need to set up an evaluation framework and systematically experiment your way to high quality results (Ragas & LlamaIndex are two evaluation approaches we’ve used most recently). Without this, you will just end up playing whack-a-mole - which is admittedly what we did at first. But learning by doing is exactly why we act as guinea pigs for our own offering :)
Real-life AI adventures with our clients
FutuCortex was our first foray into generative AI and sales, but we’ve since been working on this topic with our clients across media, transportation and manufacturing.
Throughout, the topic of how to reuse knowledge & expertise in solution selling has continued to be relevant. But we’ve also stretched into new areas:
- Automating Tender/RFP processing: in many domains, a lot of manual work goes into turning long requests of 10s or 100s of pages into a proposal. LLMs can be used in conjunction with machine learning models (like classifiers) to extract the key information from these long documents, and structure or summarise it into whatever form is needed to make it easy for your systems and people to take action. We can then take that key information and match it with the best fitting products and most similar past proposals. So we can go from super long tender to key information + recommended products & knowledge assets automatically. With this, we can take a weeks-long process down days.
- AI-based product configuration: in manufacturing, configuring products to meet client needs can be a very manual process, led by senior sales engineers who are in limited supply. With optimisation algorithms and LLMs, we can now create AI solutions that even outperform human designs. This opens the possibility to turn physical sales to digital and scale much faster.
- Personalisation in digital sales: in outreach, business leaders have been getting more and more noise in their inboxes & DMs - so how to stand out? By feeding LLMs with information on both the client’s context and your own knowledge, they can generate personalised messages that repurpose your own knowledge for a specific client. And you can fine-tune the model to ensure it does so while capturing your unique tone of voice.
Rethink your sales process from end to end
Individual use cases are where the thinking & learning should start. But with each solution, you build the tech foundation, understanding, buy-in and momentum for more.
So, as you progress, the perspective should be; how can we rethink our sales process from end to end? Eventually, the goal should be to have a clear vision for your process that all your use cases are driving towards - and overarching metrics that enable you to track the progress (e.g. reduce sales cycle time by X%, or increase self-service or digital sales by Y% ).
We’ve now seen the impact first hand from outreach, to tender processing, to proposal creation, contracting & sourcing. And this is a paradigm shift no doubt. So get moving!
- Jack RichardsonData & AI Transformation Lead